This is A Call for Fairness and Diversity Within The AI Generative Sector
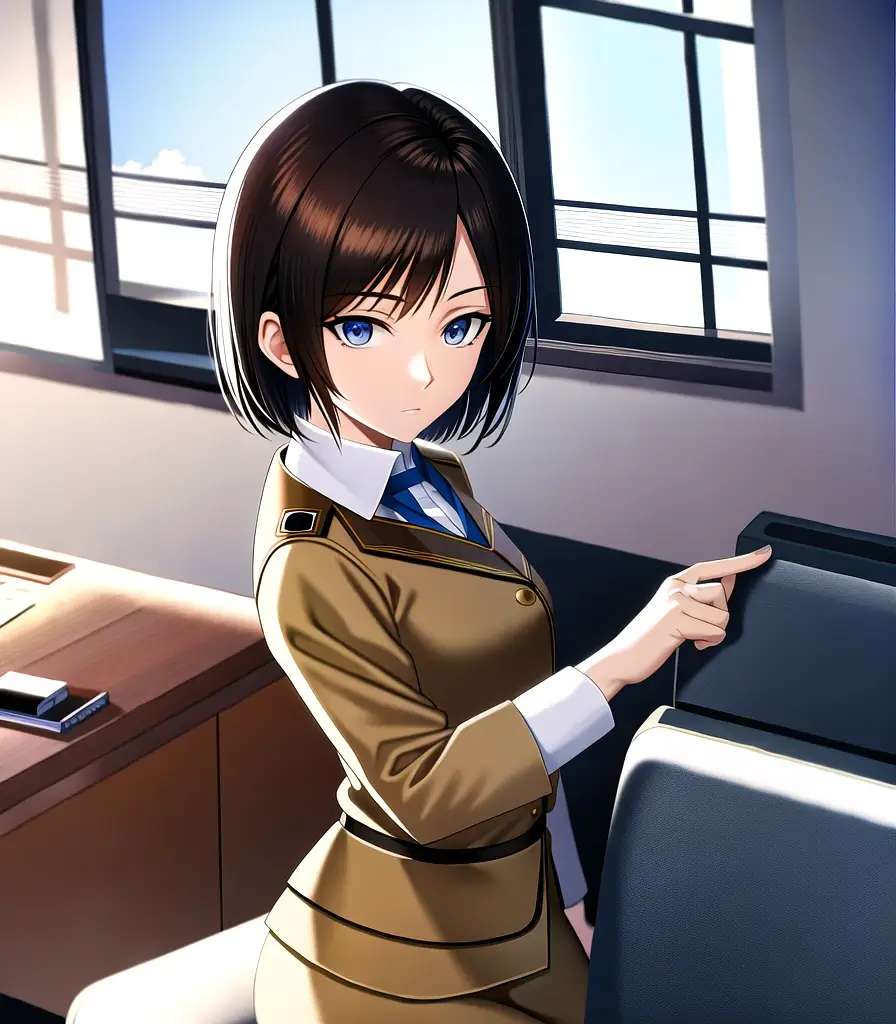
Artificial intelligence (AI) has opened up exciting possibilities in the realm of image generation, where machines can create lifelike and diverse visuals from text descriptions. However, a growing concern arises as studies reveal that AI image generators may not be immune to bias and discrimination.
Prominent models like Midjourney, Stable diffusion and DALL-E 2 (bing image create) have been found to perpetuate racial and gender stereotypes, reflecting the limitations of their algorithms and training data. This has caused a lot of concern within the AI community.
AI image generators function through machine learning models that interpret text inputs and produce corresponding images. These models rely on massive datasets containing millions of images, which they analyze to discern patterns and features.
The diffusion technique is a common method, where random noise is added to images, and the model learns to progressively eliminate it, eventually obtaining a clear image. But a lot of people are asking questions about the images used and how this new AI technology creates the images it does.
What Causes These Kind of Biases?
Well there are several factors that contribute to the bias observed in these AI image generation programs. Here is a few of the issues we found:
Algorithmic design
Implicit assumptions or preferences within the algorithm can influence the output. For instance, certain aspects of an image, such as faces or salient objects, might be prioritized over other elements like backgrounds or details.
Data diversity
Bias can arise from skewed datasets that fail to represent the full spectrum of human diversity. For example, datasets may be imbalanced, featuring more images of white people than people of color or more images of men than women.
Input specificity
Ambiguous or vague text prompts may lead to biased outcomes. Terms like “CEO” or “journalist” may not offer enough clarity, inadvertently guiding the model towards stereotypes or defaults. This is mainly down to the models training. Unless data scientists strictly correct these issues they will continue to persist. W
We can’t just create a blanket negative prompt and hope for the best. Issues would still appear when using this method of image generation.
Some Examples Of Bias
Here are a few examples of bias in AI image generation that have been found:
Racial bias
Studies have shown that AI image generators tend to favor white people over people of color for generic terms or occupations. Midjourney and DALL-E 2 produced more images of white individuals for terms like “CEO,” “doctor,” “lawyer,” and “teacher.” One of the many dark sides AI has to offer. This is due to again the training of the models that create these images.
Gender bias
AI image generators also exhibited a preference for men over women when generating images for specific roles or professions. Terms like “news analyst,” “news commentator,” and “fact-checker” saw more male representations. This can be combatted by showing more images or other genders performing these roles.
Ageism
Another bias emerged as AI image generators leaned towards younger people and, when depicting older individuals, exclusively portrayed older men in certain roles like “journalist” or “reporter.”
Let’s face it AI image generation was trained on millions of images found online. What this bias shows is that more images of this nature were available for them to crawl. It does not mean the tool is racist or biased it is just creating images based on the data it has.
Let’s face it there are women mechanics in the world plenty of them but compared to men there is a lot less and then you have to consider how many images of this profession are online and what data the bot has collected. We can’t blame the tool for it’s creators training methods.
One way you can combat this is to be a prompt engineer and be more descriptive with your keywords so if you want a female mechanic tell the AI tool that. Or else it will assume what is more probable by the data it holds.

This Bias Can Be a Cause For Concern
Although the majority of these biases are not intended to be an attack on any group or individual some people will try to make it out like it is. So in some circumstances the implications of bias in AI image generation can be significant. Here are some examples:
Reinforcement of stereotypes
AI-generated images that echo existing biases in society can reinforce harmful stereotypes about certain groups, such as people of color, women, and older individuals.
Diminished diversity and representation
By excluding or marginalizing certain groups, AI-generated images may undermine the diversity and representation of human experiences in various domains like media, education, and art.
Influence on decision-making and behavior
AI-generated images can shape how people perceive themselves and others, subsequently influencing their decision-making and behavior in contexts like hiring, voting, dating, and shopping.
To those who are new to AI generated images they may not be aware that you can pretty much generate any image you like. So if you want an Mario wearing a bikini (no we are not going to show you) you can ask for that. AI generated art can be specific or as vague as you want it to be.
Most of these biases were found when users entered “draw me a news reporter”, “draw me a mechanic” or “draw me a teacher”. The images they got made some people angry as it can reinforce certain biases. But as we have said to people before, be specific with your prompts. Ask the bot exactly what you want.
Addressing Biases Within The AI Image Generation Sector
In order for us to stop some of these biases getting out of hand we need to address them within AI generation communites. This requires a collective effort from from multiple stakeholders:
Researchers
They must design and evaluate algorithms that embody fairness, transparency, accountability, and ethics. Collecting and curating diverse, balanced, and unbiased data is equally crucial.
Developers
Implementing and testing robust, reliable, and secure algorithms is essential. Providing comprehensive documentation, guidelines, and feedback mechanisms for users and clients will aid in responsible usage.
Users
Awareness of the potential and limitations of AI image generators is crucial. Users should exercise responsibility and respect when deploying and sharing AI-generated images.

Conclusion
AI image generation holds tremendous promise for various fields and domains, spurring creativity and innovation. You can ask an AI tool to generate any image you like, you are only limited by your imagination and your own prompt writing skills.
Nonetheless, addressing bias and discrimination is paramount, ensuring that this technology is harnessed for the greater good and not perpetuating societal injustices.
Here at So Artificial all we ask is that users who are creating AI art be specific with your prompts and don’t go out of your way to cause offensive issues that you can share with others in an attempt to discredit these AI tools.