Artificial Intelligence Shows Promise in Enhancing Alcohol Use Screening in Healthcare
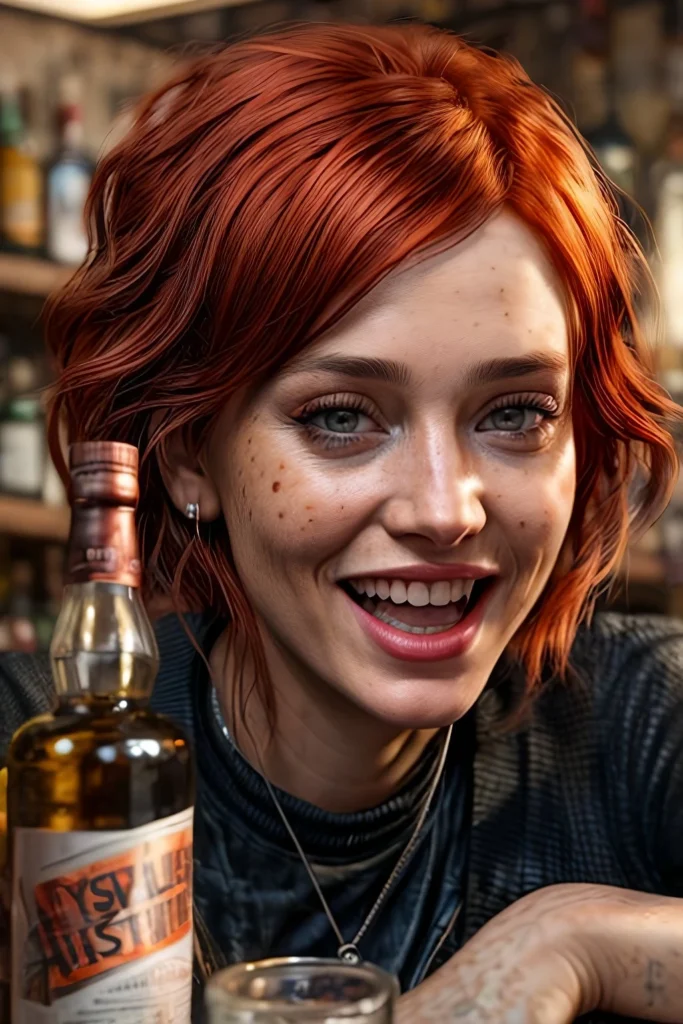
A recent study published in Alcohol: Clinical and Experimental Research suggests that an artificial intelligence-based program has demonstrated efficiency and accuracy in identifying patients with risky alcohol use by analyzing their health records. The study emphasizes the potential of AI-driven natural language processing to outperform traditional diagnostic codes in identifying individuals at risk for alcohol-related issues. This development is crucial for early intervention and preventive measures to address alcohol-related surgical complications.
Key Findings:
- Accuracy Improvement: The AI-based natural language processing algorithm showcased a notable improvement in accuracy compared to relying solely on diagnostic codes. It identified three times more patients with risky alcohol use.
- First-of-Its-Kind Approach: The study is noted as the first to leverage natural language processing, a subset of artificial intelligence, to analyze clinical text in patient records for identifying risky alcohol patterns before surgery.
- Algorithm Development and Testing: Researchers developed and tested the natural language processing algorithm by scanning clinical notes in 54,000 patient records, classifying text indicative of alcohol use disorder and risky drinking. The algorithm demonstrated a 15% positive identification rate for risky alcohol use, in contrast to the 5% identified using diagnostic codes alone.
Significance of Natural Language Processing
Traditional diagnostic codes within electronic health records often under-identify alcohol-related conditions. Clinical text provides a more comprehensive view of a patient’s history, including details about alcohol use and related problems. Natural language processing, which interprets human language, becomes essential for extracting and understanding such information from clinical text.
Study Limitations
While the study showcases promising results, researchers acknowledged limitations, including a relatively small test sample and a dataset primarily composed of White and non-Hispanic patients. The algorithm did not correct for biases based on demographic factors, raising awareness about the need for diverse representation in AI applications.
Clinical Implications
Identifying patients at risk of alcohol-related problems early is crucial, especially in the context of surgical interventions. Individuals with risky alcohol use patterns may experience higher rates of infections and complications post-surgery. The natural language processing tool developed in this study could serve as a valuable supplement to existing alcohol screening methods, aiding clinicians in making informed decisions and facilitating timely interventions.
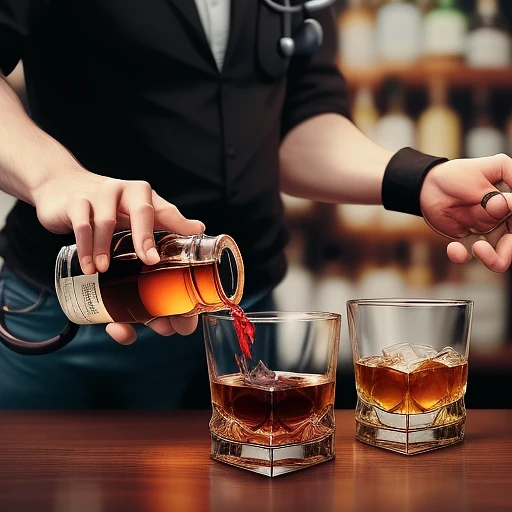
The study underscores the potential of artificial intelligence, specifically natural language processing, in enhancing alcohol use screening in healthcare settings. By improving accuracy in identifying risky alcohol patterns, AI-driven approaches contribute to early intervention and preventive strategies, aligning with the broader goal of personalized and effective patient care.